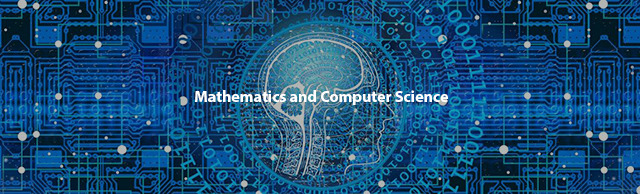
Distinguished Student Work
Document Type
Paper
Publication Date
11-14-2016
Advisor(s)
Steve Condie, Illinois Mathematics and Science Academy
Description
As technology advances and the pace of life quickens, companies are expected to continually provide better services, be more accessible, and respond to requests faster. While such expectations have led to the rapid growth of retail stores and service locations throughout the United States, delivery services have arguably been the most influenced by these rising demands.
Amazon.com is an online storefront that has seen tremendous growth throughout its lifetime, and it only continues to grow. Founded in 1994 as an online bookstore, the digital retailer eventually expanded its services to include technology, home goods, and even eBooks. Amazon’s response to its customer’s rising demands of customers was to grow and evolve. In February of 2005, Amazon launched Amazon Prime, a service that provided express shipping for hundreds of thousands of products. Amazon believed that fast deliveries should not be an occasional indulgence, but an everyday experience [1]. While the company previously advertised a four-to-six day shipping time, Amazon Prime boasts a mere two day shipping time. Prime was so popular with customers that in 2014, the electronic retailer decided to evolve even further, adding Prime Now, a same day delivery option, to its list of services, granting members free two hour delivery.
Prime Now, PrimeNow.com, and Amazon’s constant growth makes one wonder, "How can Amazon achieve such absurdly short delivery times?" A likely answer lies with efficiently distributed warehouses.
While it is possible for a delivery service company to operate solely from its headquarters, such centralization would result in inconsistent, sub-optimal delivery times, with times differing between locations nearer and farther away. The solution is to have warehouses scattered throughout the country, ensuring the presence of nearby locations to service any given customer. On the same note, it is important to consider the physical viability of such an option. A warehouse can be expensive to maintain, so the amount and placement of the warehouses must be optimized such that the service requirement can be met while also being cost efficient. Companies like Amazon who can afford to offer free delivery succeeded in balancing coverage area and company costs, and our company, SportsmanSHIP, intends to do the same.
SportsmanSHIP is a recreational equipment company based in New Hampshire. Currently, we have a physical location that customers can buy from, but most of our business is conducted through online sales. Due to an increased demand for our products, we have decided to expand our company by opening warehouses across America. Amazon currently has great coverage across the United States, guaranteeing two day shipping for all areas, and two hour shipping for select locations, but we believe that we can do better. We are a team of SportsmanSHIP employees that also share a passion for mathematical modeling, and we have been tasked with optimizing SportsmanSHIP’s warehouse locations. Our warehouse distribution will allow us to provide one day shipping to nearly all of the United States, and we will do so with minimal warehouses.
In this paper, we analyze the crux of large delivery service companies: efficient warehouse distribution. We identify important factors of efficiency such as amount of warehouses, population density, and national coverage. Using these factors, along with data given in regional maps, we constructed a comprehensive model for efficient warehouse placement. Our ultimate goal was to lower shipping time below one day for customers across the United States while minimizing the number of warehouses. In addition, we were asked to consider and discuss the implications of state sales tax on the model, as well as to account for taxes on clothing and apparel, which SportsmanSHIP plans on adding to our inventory in the near future.
To tackle the task at hand, we implemented a model that used a Markov Chain Monte Carlo (MCMC) algorithm to determine the utility of a sample distribution. For the first task, maximizing Page 2 of 26 Team #7204 national coverage and minimizing number of warehouses, this utility function considered in the percentage of the United Stat;es covered by a one-day warehouse shipping facility as well as the number of warehouses used. For the next task, we extended this model to include a sales tax calculation factor, negatively impacting the utility of a model with a large amounts of sales tax. For the third task, we modified the sales tax factor to include a weighted tax factor that took into account both clothing and sales taxes. Finally, we developed a separate economic model that compares utility based off of profit only, and does not weight the percent of the United States covered nearly as strongly. This was implemented as another way to look at the problem of optimizing warehouses, since it has been found that only 4% of customers truly care about same-day or one-day shipping [10].
Using our models, SportsmanSHIP’s expansion is guaranteed to be a success, and one day, we may even surpass Amazon. All it takes is a bit of warehouse knowledge, and a lot of math.
Recommended Citation
Agarwal, Ankit '17; Tong, William '17; Lou, Robert '17; and Moe, George '17, "2016: "Maximizing Customer Convenience with Hill Climbing"" (2016). Distinguished Student Work. 4.
https://digitalcommons.imsa.edu/math_sw/4
Comments
The team was awarded National Finalist at the HiMCM International Mathematics Modeling Contest.
Of the 840 teams entered, only 9 teams achieved this designation.