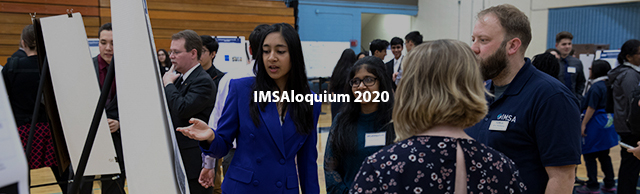
IMSA CMS - Feldman Cousins
Session Number
Project ID: PHYS 06
Advisor(s)
Dr. Peter Dong; llinois Mathematics and Science Academy
Dr. Leonard Spiegel; Fermilab
Discipline
Physical Science
Start Date
22-4-2020 8:50 AM
End Date
22-4-2020 9:05 AM
Abstract
The Feldman-Cousins method was used in this analysis to perform a frequentist analysis which can both provide frequentist coverage for the Bayesian limit and provide another limit to compare to the Bayesian one. The Feldman-Cousins approach unifies upper confidence limits and two-sided confidence intervals. This solves the problem that a prior choice of upper limit or two-sided interval leads to intervals that are not confidence intervals if the choice is dependent on the data. The Feldman-Cousins approach involves sorting Monte-Carlo generated pseudoexperiments by R-value (or likelihood ratio). In the single channel case, this is equivalent to sorting by the generated values, but in the general N-bin case, this involves sorting a number of points in N dimensional space by their R-values. After removing the points with the lowest likelihood, the remaining points define a region in space; from here, it must be determined whether a given point corresponding to the observed value is contained in the defined region. Using the current "box" approximation, a lower limit of 15.47 TeV was found compared to the Bayesian limit of 21.14 TeV. A multi-dimensional convex hull algorithm will be used to raise this limit even further. A convex hull is a vast improvement on a box as it has a much smaller volume which might contain the observed event. This means that the point at which the observed particle enters the region is likely a higher value then a box would approximate, setting a much higher lower limit. The convex hull is implemented via the QuickHull algorithm which generates all of the planes of the convex hull by generating planes to the furthest point recursively. This algorithm has never been implemented for a general n-dimensional case, and once fully implemented will likely increase the frequentist lower limit, perhaps even higher than the Bayesian limit.
IMSA CMS - Feldman Cousins
The Feldman-Cousins method was used in this analysis to perform a frequentist analysis which can both provide frequentist coverage for the Bayesian limit and provide another limit to compare to the Bayesian one. The Feldman-Cousins approach unifies upper confidence limits and two-sided confidence intervals. This solves the problem that a prior choice of upper limit or two-sided interval leads to intervals that are not confidence intervals if the choice is dependent on the data. The Feldman-Cousins approach involves sorting Monte-Carlo generated pseudoexperiments by R-value (or likelihood ratio). In the single channel case, this is equivalent to sorting by the generated values, but in the general N-bin case, this involves sorting a number of points in N dimensional space by their R-values. After removing the points with the lowest likelihood, the remaining points define a region in space; from here, it must be determined whether a given point corresponding to the observed value is contained in the defined region. Using the current "box" approximation, a lower limit of 15.47 TeV was found compared to the Bayesian limit of 21.14 TeV. A multi-dimensional convex hull algorithm will be used to raise this limit even further. A convex hull is a vast improvement on a box as it has a much smaller volume which might contain the observed event. This means that the point at which the observed particle enters the region is likely a higher value then a box would approximate, setting a much higher lower limit. The convex hull is implemented via the QuickHull algorithm which generates all of the planes of the convex hull by generating planes to the furthest point recursively. This algorithm has never been implemented for a general n-dimensional case, and once fully implemented will likely increase the frequentist lower limit, perhaps even higher than the Bayesian limit.