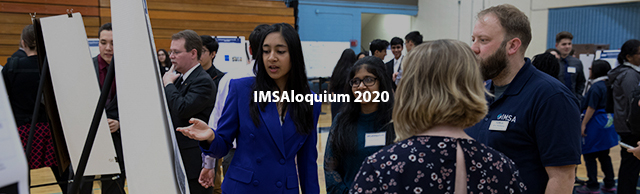
Encoder-Decoder Frameworks for Translation Between Human and Mouse Proteins
Session Number
Project ID: CMPS 4
Advisor(s)
Dr. Aly Azeem Khan; University of Chicago
Discipline
Computer Science
Start Date
22-4-2020 10:05 AM
End Date
22-4-2020 10:20 AM
Abstract
Over the last few years, increased computational power from technologies such as CUDA has allowed data scientists to build larger and larger models. One area that has significantly benefited from these improvements is machine translation, where models based on deep neural networks have replaced more traditional statistical techniques due to encoder-decoder methods. We attempt to apply these translation methods in an immunology context, where often reactions between proteins are tested in model organisms such as mice instead of humans, and therefore being able to “translate” between mouse proteins and their human analogues can help predict whether the same reactions will occur. We test various convolutional and RNN encoder-decoder frameworks on this task, and replace the linguistically-inspired translation accuracy metrics with biologically-inspired protein similarity metrics. While our models still clearly need improvements, our preliminary results represent a first step towards this ability.
Encoder-Decoder Frameworks for Translation Between Human and Mouse Proteins
Over the last few years, increased computational power from technologies such as CUDA has allowed data scientists to build larger and larger models. One area that has significantly benefited from these improvements is machine translation, where models based on deep neural networks have replaced more traditional statistical techniques due to encoder-decoder methods. We attempt to apply these translation methods in an immunology context, where often reactions between proteins are tested in model organisms such as mice instead of humans, and therefore being able to “translate” between mouse proteins and their human analogues can help predict whether the same reactions will occur. We test various convolutional and RNN encoder-decoder frameworks on this task, and replace the linguistically-inspired translation accuracy metrics with biologically-inspired protein similarity metrics. While our models still clearly need improvements, our preliminary results represent a first step towards this ability.