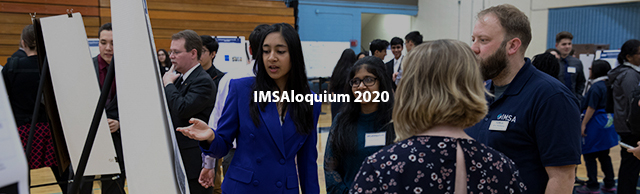
Gravitational Lensing with Generative Adversarial Networks
Session Number
Project ID: PHYS 17
Advisor(s)
Dr. Brian Nord; Fermilab, University of Chicago
Discipline
Physical Science
Start Date
22-4-2020 10:25 AM
End Date
22-4-2020 10:40 AM
Abstract
We present a new method to simulate gravitational lensing using model-assisted generative adversarial networks (MAGAN) developed by Alonso-Monsalve and Whitehead (2018). The MAGAN is trained to emulate Lenstronomy simulations created by Birrer et. al (2015). The network model is used to save time in generating large datasets of gravitational lensing. MAGANs are neural networks with parameter inputs to target specific features that the network should generate. Our research shows the feasibility of this method and an analysis of the accuracy of our MAGAN after certain training steps, comparing its training and run time to Lenstronomy. The majority of our training time is spent simulating images in order to train the neural network, and lies in the ray tracing step of the Lenstronomy package, placing a bottleneck on accuracy with lower training iterations possible in a given amount of time. The trade-off in training time does result in progressively more accurate images produced by the MAGAN, and at a faster runtime than Lesntronomy.
Gravitational Lensing with Generative Adversarial Networks
We present a new method to simulate gravitational lensing using model-assisted generative adversarial networks (MAGAN) developed by Alonso-Monsalve and Whitehead (2018). The MAGAN is trained to emulate Lenstronomy simulations created by Birrer et. al (2015). The network model is used to save time in generating large datasets of gravitational lensing. MAGANs are neural networks with parameter inputs to target specific features that the network should generate. Our research shows the feasibility of this method and an analysis of the accuracy of our MAGAN after certain training steps, comparing its training and run time to Lenstronomy. The majority of our training time is spent simulating images in order to train the neural network, and lies in the ray tracing step of the Lenstronomy package, placing a bottleneck on accuracy with lower training iterations possible in a given amount of time. The trade-off in training time does result in progressively more accurate images produced by the MAGAN, and at a faster runtime than Lesntronomy.