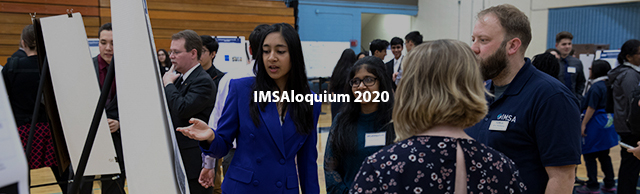
Detecting Ghosting Artifacts in Telescope Images using Machine Learning
Session Number
Project ID: ERSP 3
Loading...
Advisor(s)
Dr. Chihway Chang; University of Chicago, Fermilab
Discipline
Earth and Space Sciences
Start Date
22-4-2020 11:30 AM
End Date
22-4-2020 11:55 AM
Abstract
When unwanted artifacts appear in telescope images, whether through imperfect optics, faulty image sensors, cosmic ray hits, or airplanes and satellites, it becomes difficult to process the images for data as the artifacts can confuse data-processing programs. An artifact that is especially difficult to avoid is the ghosting artifact or the ghost, which is caused by scattering and internal reflections of light of the telescope’s mechanical and optical components. Because large cosmological surveys collect thousands of images per night, it’s extremely time-consuming to search for and manually label the images with artifacts. In our study, we used data from the Dark Energy Survey to construct a machine learning model for distinguishing ghosts automatically. This paper details the model’s structure and training process and presents the images used for training and validation. The model’s performance was compared with that of the ray-tracing program currently being used for distinguishing ghosts.
Creative Commons License
This work is licensed under a Creative Commons Attribution-NonCommercial-No Derivative Works 4.0 International License.
Detecting Ghosting Artifacts in Telescope Images using Machine Learning
When unwanted artifacts appear in telescope images, whether through imperfect optics, faulty image sensors, cosmic ray hits, or airplanes and satellites, it becomes difficult to process the images for data as the artifacts can confuse data-processing programs. An artifact that is especially difficult to avoid is the ghosting artifact or the ghost, which is caused by scattering and internal reflections of light of the telescope’s mechanical and optical components. Because large cosmological surveys collect thousands of images per night, it’s extremely time-consuming to search for and manually label the images with artifacts. In our study, we used data from the Dark Energy Survey to construct a machine learning model for distinguishing ghosts automatically. This paper details the model’s structure and training process and presents the images used for training and validation. The model’s performance was compared with that of the ray-tracing program currently being used for distinguishing ghosts.