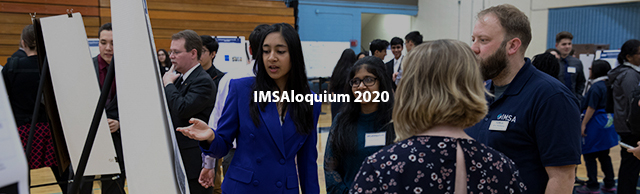
Machine learning prediction of glioblastoma patient one-year survival using clinical and genomic data
Session Number
Project ID: MEDH 32
Loading...
Advisor(s)
Dr. Warren McGee; Northwestern University Feinberg School of Medicine, Department of Neurology
Dr. Jane Wu; Northwestern University Feinberg School of Medicine, Department of Neurology
Discipline
Medical and Health Sciences
Start Date
22-4-2020 11:30 AM
End Date
22-4-2020 11:55 AM
Abstract
This study aimed to use machine learning to predict one-year survival for primary glioblastoma (pGBM) patients using data (n = 175) from the Chinese Glioma Genome Atlas (CGGA). Logistic regression (LR), support vector machine (SVM), random forest (RF), and ensemble models were used to select predictors for overall survival (OS) and to classify patients into those surviving less than one year and one year or greater.
With respect to OS, significant (p < 0.05) correlation was found with age (negative), radiotherapy (positive), and chemotherapy (positive). IDH1 mutation and 1p19q codeletion showed insignificant correlation with OS. However, IDH1 mutation showed significant negative correlation with age. Thus, further study may reveal long-term prognostic value.
Correlation analysis was performed on mRNAseq FPKM data to select for significance. LR, SVM, and RF classifiers were compared and combined in a weighted soft-voting ensemble classifier, using weights of 0.125, 0.125, and 0.750, respectively. The ensemble model had the highest accuracy (AUC = 0.654, F1 = 0.799). LR and SVM appeared to underfit the data, while RF appeared to overfit the data. In the ensemble model, the overfitting tendency of RF appeared to be counteracted by the underfitting tendencies of LR and SVM while maintaining high accuracy.
Machine learning prediction of glioblastoma patient one-year survival using clinical and genomic data
This study aimed to use machine learning to predict one-year survival for primary glioblastoma (pGBM) patients using data (n = 175) from the Chinese Glioma Genome Atlas (CGGA). Logistic regression (LR), support vector machine (SVM), random forest (RF), and ensemble models were used to select predictors for overall survival (OS) and to classify patients into those surviving less than one year and one year or greater.
With respect to OS, significant (p < 0.05) correlation was found with age (negative), radiotherapy (positive), and chemotherapy (positive). IDH1 mutation and 1p19q codeletion showed insignificant correlation with OS. However, IDH1 mutation showed significant negative correlation with age. Thus, further study may reveal long-term prognostic value.
Correlation analysis was performed on mRNAseq FPKM data to select for significance. LR, SVM, and RF classifiers were compared and combined in a weighted soft-voting ensemble classifier, using weights of 0.125, 0.125, and 0.750, respectively. The ensemble model had the highest accuracy (AUC = 0.654, F1 = 0.799). LR and SVM appeared to underfit the data, while RF appeared to overfit the data. In the ensemble model, the overfitting tendency of RF appeared to be counteracted by the underfitting tendencies of LR and SVM while maintaining high accuracy.