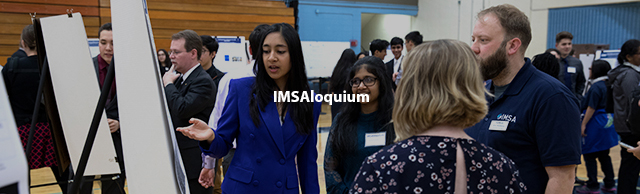
Performing Hypothesis Testing Involving Candidate Topological Spaces Generated by Polyominoes
Session Number
Project ID: MATH 02
Advisor(s)
Ryan Robinett, University of Chicago
Discipline
Mathematics
Start Date
20-4-2022 8:50 AM
End Date
20-4-2022 9:05 AM
Abstract
Topological data analysis (TDA) is an approach to the analysis of datasets using techniques from topology. The main tool is persistent homology, an adaptation of homology to point cloud data. Persistent homology has been applied to many types of data across many fields. Until quite recently, the theoretical aspects of TDA and topological inference mostly relied on deterministic approaches. These deterministic approaches do not take into account the random nature of data and the intrinsic variability of the topological quantity they infer. Consequently, most of the corresponding methods remain exploratory, without being able to efficiently distinguish between nformation and what is sometimes called "topological noise". A statistical approach to TDA means that we consider data as generated from an unknown distribution, but also that the inferred opological features by TDA methods are seen as estimators of topological quantities describing an underlying object.
Our study aims to use percolation model where (d-1) simplices are used as edges and d simplices are used as nodes from graph theory to generate polyominoes that will produce different probability distributions of persistence diagrams, so that we can perform hypothesis tests involving candidate topological spaces, and better understand how topological noise arises through different probability distributions.
Performing Hypothesis Testing Involving Candidate Topological Spaces Generated by Polyominoes
Topological data analysis (TDA) is an approach to the analysis of datasets using techniques from topology. The main tool is persistent homology, an adaptation of homology to point cloud data. Persistent homology has been applied to many types of data across many fields. Until quite recently, the theoretical aspects of TDA and topological inference mostly relied on deterministic approaches. These deterministic approaches do not take into account the random nature of data and the intrinsic variability of the topological quantity they infer. Consequently, most of the corresponding methods remain exploratory, without being able to efficiently distinguish between nformation and what is sometimes called "topological noise". A statistical approach to TDA means that we consider data as generated from an unknown distribution, but also that the inferred opological features by TDA methods are seen as estimators of topological quantities describing an underlying object.
Our study aims to use percolation model where (d-1) simplices are used as edges and d simplices are used as nodes from graph theory to generate polyominoes that will produce different probability distributions of persistence diagrams, so that we can perform hypothesis tests involving candidate topological spaces, and better understand how topological noise arises through different probability distributions.