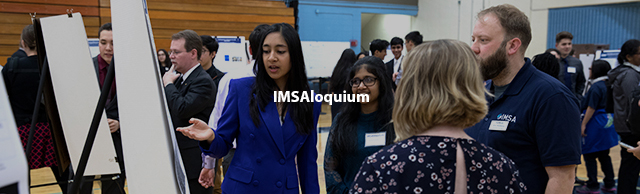
A Bayesian hierarchical model of longitudinal dynamics
Session Number
Project ID: MEDH 16
Advisor(s)
Andrew Vigotsky; Center of Translational Pain, Feinberg School of Medicine, Northwestern University
Dr. A. V. Apkarian, Center of Translational Pain, Feinberg School of Medicine, Northwestern University
Discipline
Medical and Health Sciences
Start Date
20-4-2022 11:55 AM
End Date
20-4-2022 12:20 PM
Abstract
Pain is the most ubiquitous and economically burdensome condition in the world. For this reason, pain relief—a decrease in pain over time—is the primary outcome for clinical trials across many fields, ranging from pharmaceuticals to physiotherapy. Often measured at few, discrete time points (e.g., pre- and post-intervention), measures and statistical models of pain relief are poor. The low temporal resolution increases vulnerability to regression-to-the-mean, decreases the precision of treatment effect estimates, and patient-specific trends in pain relief can neither be captured, forecasted, nor explained. Here, we propose a Bayesian hierarchical model of longitudinal pain relief that contains two Markovian states: a pain trajectory state and a pain flare state. Our model makes use of daily pain rating data to model time points within states within individuals. Group assignments can be used to investigate the effects of interventions while demographic and patient-specific parameters—such as psychometric assessments and brain properties—can be used for predictive modeling. By fully exploiting time-series data, we can obtain better estimates of treatment effects, in addition to identifying parameters that explain both between- and within-patient heterogeneity. Our model represents a step towards high- resolution patient symptom monitoring, disease progression, and individualized clinical forecasting.
A Bayesian hierarchical model of longitudinal dynamics
Pain is the most ubiquitous and economically burdensome condition in the world. For this reason, pain relief—a decrease in pain over time—is the primary outcome for clinical trials across many fields, ranging from pharmaceuticals to physiotherapy. Often measured at few, discrete time points (e.g., pre- and post-intervention), measures and statistical models of pain relief are poor. The low temporal resolution increases vulnerability to regression-to-the-mean, decreases the precision of treatment effect estimates, and patient-specific trends in pain relief can neither be captured, forecasted, nor explained. Here, we propose a Bayesian hierarchical model of longitudinal pain relief that contains two Markovian states: a pain trajectory state and a pain flare state. Our model makes use of daily pain rating data to model time points within states within individuals. Group assignments can be used to investigate the effects of interventions while demographic and patient-specific parameters—such as psychometric assessments and brain properties—can be used for predictive modeling. By fully exploiting time-series data, we can obtain better estimates of treatment effects, in addition to identifying parameters that explain both between- and within-patient heterogeneity. Our model represents a step towards high- resolution patient symptom monitoring, disease progression, and individualized clinical forecasting.