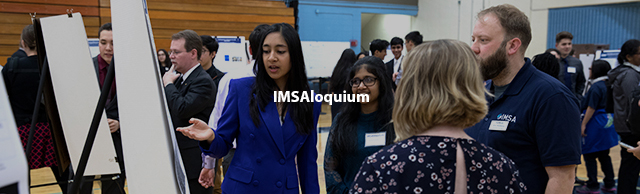
A High-throughput Machine Learning Model for Predicting Neuronal Identity and Identifying Mutation-caused Neuropathological Defects
Session Number
Project ID: CMPS 11
Advisor(s)
Jubao Duan; NorthShore University HealthSystem
Siwei Zhang; NorthShore University HealthSystem
Discipline
Computer Science
Start Date
19-4-2023 8:50 AM
End Date
19-4-2023 9:05 AM
Abstract
Congenital mental disorders, such as schizophrenia and major depressive disorder, affect approximately 20% of the US population. Research in clinical psychiatry, brain development, and medical genetics has established causal links between the nature of genetic backgrounds to the firing pattern of different types of neurons in the brain, which, in turn, leads to the psychopathological symptoms documented in clinical settings. Whilst significant efforts have been devoted to analyzing and comparing the neuronal firing patterns under different genetic contexts, such efforts are frequently complicated by low measurement throughput, neuron heterogeneity, repetitive manual and error-prone analysis, as well as categorization bias derived from different researchers. Here, we introduce a machine learning-based, fully-automated high-content imaging pipeline designed for batch-analyzing large quantities of multi-photon confocal microscope data of health and schizophrenia patient-derived neurons. Written in Python with the published and proven scientific packages CaImAn and scikit-learn, our pipeline has demonstrated abilities to perform automatic image segregation, cell identification, signal quantification, and separation of neural and non-neural (astrocytes) cells through pattern recognition, integration of training data sets, and clustering. Finally, frequency analysis revealed discrepancies in neuron firing rates from healthy and schizophrenia-risk mutant individuals. We propose our pipeline especially advantageously for mixed-culture systems.
A High-throughput Machine Learning Model for Predicting Neuronal Identity and Identifying Mutation-caused Neuropathological Defects
Congenital mental disorders, such as schizophrenia and major depressive disorder, affect approximately 20% of the US population. Research in clinical psychiatry, brain development, and medical genetics has established causal links between the nature of genetic backgrounds to the firing pattern of different types of neurons in the brain, which, in turn, leads to the psychopathological symptoms documented in clinical settings. Whilst significant efforts have been devoted to analyzing and comparing the neuronal firing patterns under different genetic contexts, such efforts are frequently complicated by low measurement throughput, neuron heterogeneity, repetitive manual and error-prone analysis, as well as categorization bias derived from different researchers. Here, we introduce a machine learning-based, fully-automated high-content imaging pipeline designed for batch-analyzing large quantities of multi-photon confocal microscope data of health and schizophrenia patient-derived neurons. Written in Python with the published and proven scientific packages CaImAn and scikit-learn, our pipeline has demonstrated abilities to perform automatic image segregation, cell identification, signal quantification, and separation of neural and non-neural (astrocytes) cells through pattern recognition, integration of training data sets, and clustering. Finally, frequency analysis revealed discrepancies in neuron firing rates from healthy and schizophrenia-risk mutant individuals. We propose our pipeline especially advantageously for mixed-culture systems.