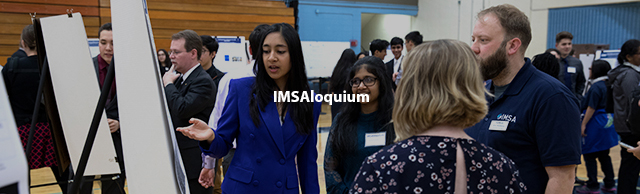
Assessing the Performance of Automated Human Phenotype Ontology (HPO) Term Extraction for Deep Phenotyping of Patients Receiving Whole Genome/Whole Exome Sequencing in a Clinical Diagnostic Laboratory
Session Number
Project ID: MEDH 10
Advisor(s)
Dr. Kai Lee Yap; Ann & Robert H. Lurie Children's Outpatient Center
Discipline
Medical and Health Sciences
Start Date
19-4-2023 8:50 AM
End Date
19-4-2023 9:05 AM
Abstract
A comprehensive list of Human Phenotype Ontology (HPO) terms capturing a patient’s phenotypic features is essential for the creation of a prioritized gene list for whole exome and whole genome sequencing (WES/WGS) analysis. However, the conversion of a patient’s clinical notes into HPO terms is inefficient, requiring human intervention, and introduces subjectivity. In this study, we evaluated the performance of various Natural Language Processing (NLP) and gene-ranking algorithms that partially automate the identification of HPO terms from rich narrative notes and accomplish deep phenotyping. manually curated a set of 50 patients who underwent WES with resultant disease-causing variants. These clinical notes were processed in the EHR-Phenolyzer pipeline, which utilizes HPO terms extracted by MetaMap to generate a ranked gene list on the Phenolyzer algorithm. The accuracy of the extracted HPO terms were compared to provider submitted terms. A cross comparison was also made with ClinPhen-Phen2Gene workflow. The EHR-Phenolyzer pipeline ranked genes with disease-causing variants at positions <250th in 38% of the WES cases (19/50), as compared to 30% of cases (15/50) using MetaMap-Phen2Gene. ClinPhen-Phen2Gene workflow demonstrated comparable performance. Adoption of NLP-assisted deep phenotyping and gene-ranking is critical to minimize the variable effects of human recall bias.
Assessing the Performance of Automated Human Phenotype Ontology (HPO) Term Extraction for Deep Phenotyping of Patients Receiving Whole Genome/Whole Exome Sequencing in a Clinical Diagnostic Laboratory
A comprehensive list of Human Phenotype Ontology (HPO) terms capturing a patient’s phenotypic features is essential for the creation of a prioritized gene list for whole exome and whole genome sequencing (WES/WGS) analysis. However, the conversion of a patient’s clinical notes into HPO terms is inefficient, requiring human intervention, and introduces subjectivity. In this study, we evaluated the performance of various Natural Language Processing (NLP) and gene-ranking algorithms that partially automate the identification of HPO terms from rich narrative notes and accomplish deep phenotyping. manually curated a set of 50 patients who underwent WES with resultant disease-causing variants. These clinical notes were processed in the EHR-Phenolyzer pipeline, which utilizes HPO terms extracted by MetaMap to generate a ranked gene list on the Phenolyzer algorithm. The accuracy of the extracted HPO terms were compared to provider submitted terms. A cross comparison was also made with ClinPhen-Phen2Gene workflow. The EHR-Phenolyzer pipeline ranked genes with disease-causing variants at positions <250th in>38% of the WES cases (19/50), as compared to 30% of cases (15/50) using MetaMap-Phen2Gene. ClinPhen-Phen2Gene workflow demonstrated comparable performance. Adoption of NLP-assisted deep phenotyping and gene-ranking is critical to minimize the variable effects of human recall bias.