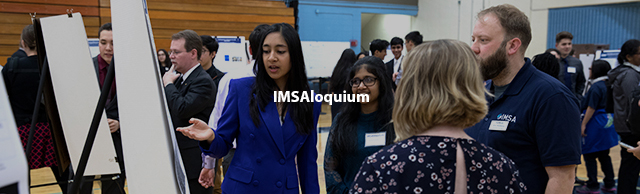
Approximating the Row-Wise Weighted Total Least Squares Regression Solution
Session Number
Project ID: MATH 01
Advisor(s)
Dr. Evan Glazer; Illinois Mathematics and Science Academy
Dr. Aritra Dutta; University of Southern Denmark
Discipline
Mathematics
Start Date
19-4-2023 9:05 AM
End Date
19-4-2023 9:20 AM
Abstract
Motivated by applications as a kernel of nonlinear regression algorithms, the row-wise weighted total least squares regression problem is examined to find a consistent and accurate estimator. Specifically, the proposed estimator has a time complexity linear in the number of observations and a space complexity constant in the same value, as the number of observations can be quite large in many modern applications, often many orders of magnitude larger than the number of input and output features. Further, to accommodate large data sets, an algorithm functions by updating an intermediate representation from each observation, allowing for parallelization of the necessary computation. Several related algorithms are proposed, based on approximating the noncentral second moment of the underlying data by a weighted mean, requiring only linear time in the number of observations. Experimental findings show the proposed algorithm to be competitive with existing methods intended to solve other variants of the Total Least Squares problem. Directions for continued iteration and further investigation are proposed.
Approximating the Row-Wise Weighted Total Least Squares Regression Solution
Motivated by applications as a kernel of nonlinear regression algorithms, the row-wise weighted total least squares regression problem is examined to find a consistent and accurate estimator. Specifically, the proposed estimator has a time complexity linear in the number of observations and a space complexity constant in the same value, as the number of observations can be quite large in many modern applications, often many orders of magnitude larger than the number of input and output features. Further, to accommodate large data sets, an algorithm functions by updating an intermediate representation from each observation, allowing for parallelization of the necessary computation. Several related algorithms are proposed, based on approximating the noncentral second moment of the underlying data by a weighted mean, requiring only linear time in the number of observations. Experimental findings show the proposed algorithm to be competitive with existing methods intended to solve other variants of the Total Least Squares problem. Directions for continued iteration and further investigation are proposed.