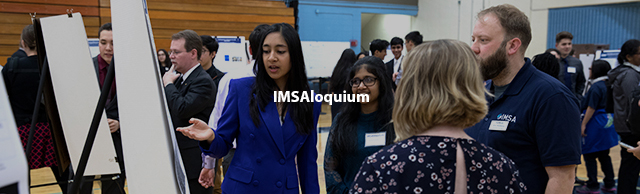
Applying Privacy-Preserving Federated Learning to Biomedical Datasets
Session Number
Project ID: CMPS 05
Advisor(s)
Dr. Ravi K. Madduri; Argonne National Laboratory
Discipline
Computer Science
Start Date
19-4-2023 9:20 AM
End Date
19-4-2023 9:35 AM
Abstract
Machine learning and artificial intelligence have become increasingly used in the healthcare industry, but they have privacy concerns. Federated learning is presented as a potential solution to these concerns, but its vulnerability to inference attacks is noted. The Argonne Privacy-Preserving Federated Learning (APPFL) framework is introduced as a privacy-preserving technique for federated learning. The project aims to evaluate the effectiveness and accuracy of training machine learning models using APPFL on biomedical datasets and electronic health record data in a simulated environment. We successfully trained models on synthetic data using a simulated instance of APPFL on one machine. The models had high accuracy, and they showed better generalization for the test set than models trained without APPFL. This project contributes to the growing literature on the use of federated learning and privacy-preserving techniques in protecting sensitive data.
Applying Privacy-Preserving Federated Learning to Biomedical Datasets
Machine learning and artificial intelligence have become increasingly used in the healthcare industry, but they have privacy concerns. Federated learning is presented as a potential solution to these concerns, but its vulnerability to inference attacks is noted. The Argonne Privacy-Preserving Federated Learning (APPFL) framework is introduced as a privacy-preserving technique for federated learning. The project aims to evaluate the effectiveness and accuracy of training machine learning models using APPFL on biomedical datasets and electronic health record data in a simulated environment. We successfully trained models on synthetic data using a simulated instance of APPFL on one machine. The models had high accuracy, and they showed better generalization for the test set than models trained without APPFL. This project contributes to the growing literature on the use of federated learning and privacy-preserving techniques in protecting sensitive data.