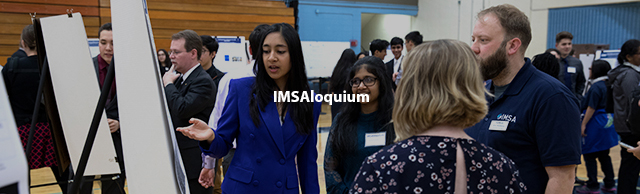
Effectiveness of Machine Learning Applications in Environmental Engineering
Session Number
Project ID: ENVR 02
Discipline
Environmental Science
Start Date
19-4-2023 10:05 AM
End Date
19-4-2023 10:20 AM
Abstract
The use of machine learning (ML) algorithms to test effectiveness and expand applications in environmental engineering has become increasingly successful. ML algorithms have the advantage of being able to address nonlinear data successfully. Furthermore, environmental engineering has become increasingly data intensive, a challenge that has been met by rapid progress in the development of computational techniques for handling large data sets. Therefore, it is often feasible to create an ML model with adequate accuracy despite the largely variable sample size of data used in energy and environmental engineering. In this project, we explore the effectiveness of different ML techniques in replacing more conventional computational methods through applications in ongoing environmental engineering projects. Accuracy varied across projects depending on factors including the characteristics of the data and types of ML modeling techniques used.
Effectiveness of Machine Learning Applications in Environmental Engineering
The use of machine learning (ML) algorithms to test effectiveness and expand applications in environmental engineering has become increasingly successful. ML algorithms have the advantage of being able to address nonlinear data successfully. Furthermore, environmental engineering has become increasingly data intensive, a challenge that has been met by rapid progress in the development of computational techniques for handling large data sets. Therefore, it is often feasible to create an ML model with adequate accuracy despite the largely variable sample size of data used in energy and environmental engineering. In this project, we explore the effectiveness of different ML techniques in replacing more conventional computational methods through applications in ongoing environmental engineering projects. Accuracy varied across projects depending on factors including the characteristics of the data and types of ML modeling techniques used.