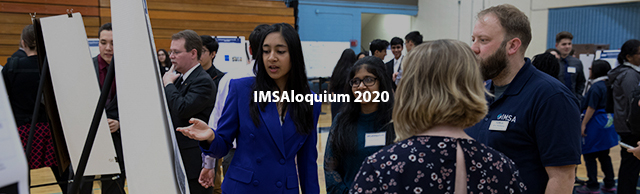
Computational Approaches to Detection of Mosaic Variants in Patients
Session Number
Project ID: BIO 03
Advisor(s)
Dr. Gemma L. Carvill; Department of Neurology, Northwestern University Feinberg School of Medicine
Jonathan Gunti; Department of Neurology, Northwestern University Feinberg School of Medicine
Discipline
Biology
Start Date
22-4-2020 8:30 AM
End Date
22-4-2020 8:45 AM
Abstract
In recent years, many bioinformatics pipelines have been developed to call variants from next generation sequencing (NGS) data for conditions like epilepsy. Epilepsy is defined by recurrent, unprovoked seizures due to excessive, hypersynchronous brain activity with 70-80% of cases likely being caused by genetic variants. Somatic variants are low-allelic-fraction mutations occurring in only a subset of cells because of either tumor heterogeneity or somatic mosaicism, the co-existence of two genetically distinct cell populations within an individual. Given these variants’ low read counts in sequenced DNA, they are often missed. Therefore, here we compare the sensitivity and specificity of two mosaic variant callers, MuTect and Mosaic Forecast, to develop the most accurate variant identification method.
Using the BWA aligner, we built two multiple-sample pipelines and applied them to whole exome sequencing (WES) data of a cohort of 21 epilepsy patients collected for a national and international collaboration. The candidate somatic variants detected using these methods were then validated with PCR amplification and Sanger sequencing or targeted NGS of the patients’ DNA samples. In our initial analysis of the cohort, MuTect identified candidate somatic variants in the genes GRK5 (p.V247A) and GLUL (p.M1V), which are currently being validated. Using BamSurgeon, we also inserted 18 simulated variants to the WES data of 16 tumor-normal pairs, and MuTect characterized 17 of the 18 simulated variants indicating high sensitivity. Our long-term goal is to develop a pipeline that detects somatic variants as a function of sequencing depth and allelic fraction to provide more informed clinical diagnostics affecting epilepsy patient treatment and outcomes.
Computational Approaches to Detection of Mosaic Variants in Patients
In recent years, many bioinformatics pipelines have been developed to call variants from next generation sequencing (NGS) data for conditions like epilepsy. Epilepsy is defined by recurrent, unprovoked seizures due to excessive, hypersynchronous brain activity with 70-80% of cases likely being caused by genetic variants. Somatic variants are low-allelic-fraction mutations occurring in only a subset of cells because of either tumor heterogeneity or somatic mosaicism, the co-existence of two genetically distinct cell populations within an individual. Given these variants’ low read counts in sequenced DNA, they are often missed. Therefore, here we compare the sensitivity and specificity of two mosaic variant callers, MuTect and Mosaic Forecast, to develop the most accurate variant identification method.
Using the BWA aligner, we built two multiple-sample pipelines and applied them to whole exome sequencing (WES) data of a cohort of 21 epilepsy patients collected for a national and international collaboration. The candidate somatic variants detected using these methods were then validated with PCR amplification and Sanger sequencing or targeted NGS of the patients’ DNA samples. In our initial analysis of the cohort, MuTect identified candidate somatic variants in the genes GRK5 (p.V247A) and GLUL (p.M1V), which are currently being validated. Using BamSurgeon, we also inserted 18 simulated variants to the WES data of 16 tumor-normal pairs, and MuTect characterized 17 of the 18 simulated variants indicating high sensitivity. Our long-term goal is to develop a pipeline that detects somatic variants as a function of sequencing depth and allelic fraction to provide more informed clinical diagnostics affecting epilepsy patient treatment and outcomes.