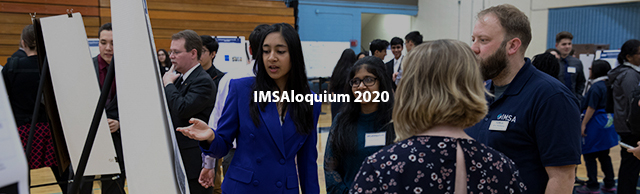
Effects of Hypertension and Diabetes in the Chicagoland Area
Session Number
Project ID: CMPS 5
Advisor(s)
Dr. Marynia Kolak; University of Chicago
Discipline
Computer Science
Start Date
22-4-2020 10:25 AM
End Date
22-4-2020 10:40 AM
Abstract
The Social Determinants of Health are economic and social factors that significantly influence individuals’ health and lifestyles. In this study, we look for correlations between SDOH data at the census tract level and patient data about hypertension and diabetes between 2012-2017 in the Chicagoland area. We identified patients with hypertension through their ICD diagnosis code, antihypertensive prescriptions, and median blood pressure values by year. Likewise, patients with diabetes were identified through their ICD diagnosis code, diabetes medication prescription, median blood glucose level by year, and median A1C level by year. We ran spatial cluster analyses to assign LISA (local indicators of spatial association) categories for each year for each disease. These categories are used to identify hotspots, clusters, and outliers. We found that prevalence rates for patients with hypertension and diabetes had a strong correlation with high rates of disability status, minority population, and home ownership. In Chicago’s South Side, high foreclosure risk was found to be associated with high rates of hypertension and diabetes. Using spatial analyses to identify relationships between disease rates and SDOH data, can reveal clusters and populations in need of care, which can be used for policy change, healthcare distribution, and future work.
Effects of Hypertension and Diabetes in the Chicagoland Area
The Social Determinants of Health are economic and social factors that significantly influence individuals’ health and lifestyles. In this study, we look for correlations between SDOH data at the census tract level and patient data about hypertension and diabetes between 2012-2017 in the Chicagoland area. We identified patients with hypertension through their ICD diagnosis code, antihypertensive prescriptions, and median blood pressure values by year. Likewise, patients with diabetes were identified through their ICD diagnosis code, diabetes medication prescription, median blood glucose level by year, and median A1C level by year. We ran spatial cluster analyses to assign LISA (local indicators of spatial association) categories for each year for each disease. These categories are used to identify hotspots, clusters, and outliers. We found that prevalence rates for patients with hypertension and diabetes had a strong correlation with high rates of disability status, minority population, and home ownership. In Chicago’s South Side, high foreclosure risk was found to be associated with high rates of hypertension and diabetes. Using spatial analyses to identify relationships between disease rates and SDOH data, can reveal clusters and populations in need of care, which can be used for policy change, healthcare distribution, and future work.