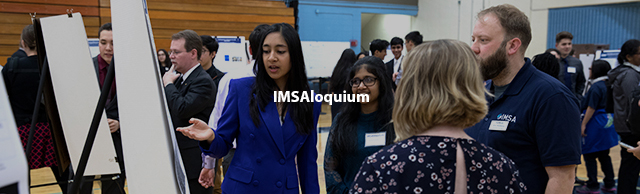
Using Social Network Analysis to Build Human-Autonomy Teams
Session Number
Project ID: BHVSO 01
Advisor(s)
Noshir Contractor; Northwestern University
Vsevolod Suschevskiy; Northwestern University
Discipline
Behavioral and Social Sciences
Start Date
19-4-2023 8:50 AM
End Date
19-4-2023 9:05 AM
Abstract
With the rise of Artificial Intelligence (AI), research must be conducted on how compatible human-autonomy teams truly are. This relationship can be measured qualitatively through a social network analysis of interactions between groups of up to nine humans and one robot, named Vero, working collaboratively to complete practical and creative tasks under a time constraint. Network ties are drawn based on the order of speaking as recorded by transcript data; thus tie strength increases with repeated interaction. ERGM coefficient for race homophily—the preference for associating with individuals of the same racial background—will define how much weight race holds in team success by representing the change in the likelihood of a tie for a unit change in a predictor. By exploring different types of homophily: race, education, gender, and socioeconomic status, the ERGM coefficients and their respective SNA model types reveal what teammate qualities, both in humans and AI models, can best predict positive team dynamics and/or team success.
Using Social Network Analysis to Build Human-Autonomy Teams
With the rise of Artificial Intelligence (AI), research must be conducted on how compatible human-autonomy teams truly are. This relationship can be measured qualitatively through a social network analysis of interactions between groups of up to nine humans and one robot, named Vero, working collaboratively to complete practical and creative tasks under a time constraint. Network ties are drawn based on the order of speaking as recorded by transcript data; thus tie strength increases with repeated interaction. ERGM coefficient for race homophily—the preference for associating with individuals of the same racial background—will define how much weight race holds in team success by representing the change in the likelihood of a tie for a unit change in a predictor. By exploring different types of homophily: race, education, gender, and socioeconomic status, the ERGM coefficients and their respective SNA model types reveal what teammate qualities, both in humans and AI models, can best predict positive team dynamics and/or team success.