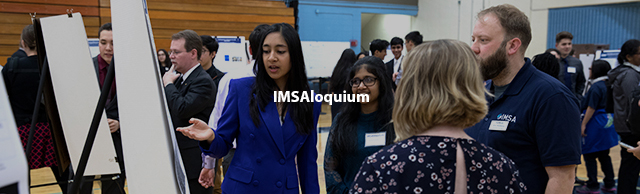
Machine Learning Diagnosis of Chronic Rhinosinusitis
Session Number
Project ID: CMPS 14
Advisor(s)
Dr. Claus-Peter Richter; Northwestern University, Feinberg School of Medicine / Department of Otolaryngology
Discipline
Computer Science
Start Date
19-4-2023 10:50 AM
End Date
19-4-2023 11:05 AM
Abstract
Chronic Rhinosinusitis (CRS) is a nasal disease characterized by inflamed mucosa and paranasal sinuses for at least 12 consecutive weeks. The disease requires 12+ weeks of characteristic symptoms, nasal endoscopy, and/or a computed tomography scan which are frequently inaccessible in primary care. This project introduces an easier method to screen for CRS using a patient’s speech patterns, signal processing algorithms, and machine learning.
Signal processing algorithms were used to inform on features suitable to be fed into a machine learning algorithm. To select the most robust signal processing algorithm, 21 participants’ speech were recorded twice, first with their nose obstructed and then non-obstructed. After selecting the best signal processing algorithm, another set of 42 CRS symptomatic patients was used to test an initial trial run of the algorithm. The time waveform provided the highest accuracy of 67%, sensitivity of 77%, and specificity of 57%.
The initial trial run resulted in a 66% accuracy, 69% sensitivity, and 62% specificity. The current method acts as a baseline for future testing, showing that the time waveform extracts the most representative features for CRS diagnosis. In the future, more training data will increase the algorithm's accuracy and allow for more powerful machine-learnin approaches.
Machine Learning Diagnosis of Chronic Rhinosinusitis
Chronic Rhinosinusitis (CRS) is a nasal disease characterized by inflamed mucosa and paranasal sinuses for at least 12 consecutive weeks. The disease requires 12+ weeks of characteristic symptoms, nasal endoscopy, and/or a computed tomography scan which are frequently inaccessible in primary care. This project introduces an easier method to screen for CRS using a patient’s speech patterns, signal processing algorithms, and machine learning.
Signal processing algorithms were used to inform on features suitable to be fed into a machine learning algorithm. To select the most robust signal processing algorithm, 21 participants’ speech were recorded twice, first with their nose obstructed and then non-obstructed. After selecting the best signal processing algorithm, another set of 42 CRS symptomatic patients was used to test an initial trial run of the algorithm. The time waveform provided the highest accuracy of 67%, sensitivity of 77%, and specificity of 57%.
The initial trial run resulted in a 66% accuracy, 69% sensitivity, and 62% specificity. The current method acts as a baseline for future testing, showing that the time waveform extracts the most representative features for CRS diagnosis. In the future, more training data will increase the algorithm's accuracy and allow for more powerful machine-learnin approaches.