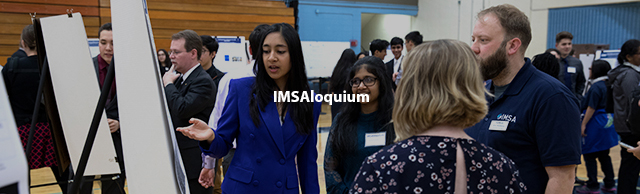
Multivariate analysis for detecting lepton jets
Session Number
Project ID: PHYS 19
Advisor(s)
Dr. Peter Dong; Illinois Mathematics and Science Academy
Discipline
Physical Science
Start Date
19-4-2023 10:50 AM
End Date
19-4-2023 11:05 AM
Abstract
Effective identification of dark photon-generated lepton jets has always been difficult to perform through standard cuts-based procedures. The possibility of solving this problem using multivariate analysis (MVA) methods has been tested. The initial list of input parameters included the total transverse momentum, azimuthal angle, pseudorapidity, number of particles, and jet width of each jet. The leading particle transverse momentum and difference in transverse momentum between the leading and runner up particles were later also found to increase model effectiveness. Various MVA classifiers (including neural networks and boosted decision trees) were trained on Monte Carlo simulations, tested on Run II data (jet width < 0.5), and validated with Run II data (jet width > 0.5). The resulting ROC curves, along with plots of the aforementioned input parameters and the distribution of model prediction on testing data is presented.
Multivariate analysis for detecting lepton jets
Effective identification of dark photon-generated lepton jets has always been difficult to perform through standard cuts-based procedures. The possibility of solving this problem using multivariate analysis (MVA) methods has been tested. The initial list of input parameters included the total transverse momentum, azimuthal angle, pseudorapidity, number of particles, and jet width of each jet. The leading particle transverse momentum and difference in transverse momentum between the leading and runner up particles were later also found to increase model effectiveness. Various MVA classifiers (including neural networks and boosted decision trees) were trained on Monte Carlo simulations, tested on Run II data (jet width < 0.5), and validated with Run II data (jet width > 0.5). The resulting ROC curves, along with plots of the aforementioned input parameters and the distribution of model prediction on testing data is presented.